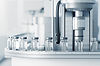
AI + Quantum:
New Solutions in Pharmacy
SUBVERT TRADITION
Tackle Industry Pain Points
Traditional pharmaceutical manufacturing faces issues like low success rates, expert dependency, high costs, and high clinical failure rates in drug discovery, in vitro activity, biological experiments, and clinical trials. Our technologies and solutions address these pain points, enhancing drug R&D efficiency and success rates.
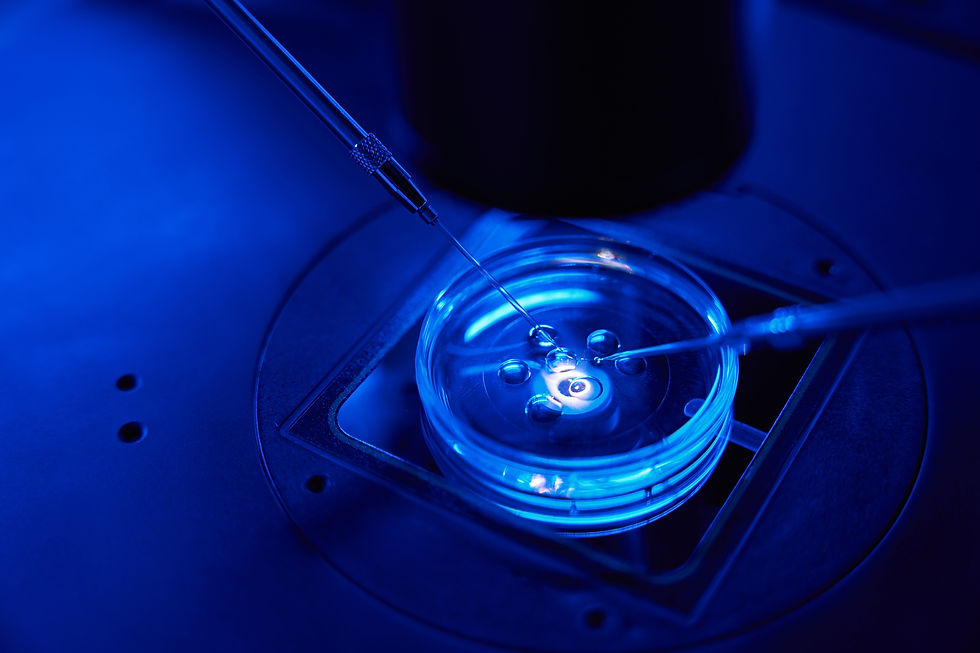
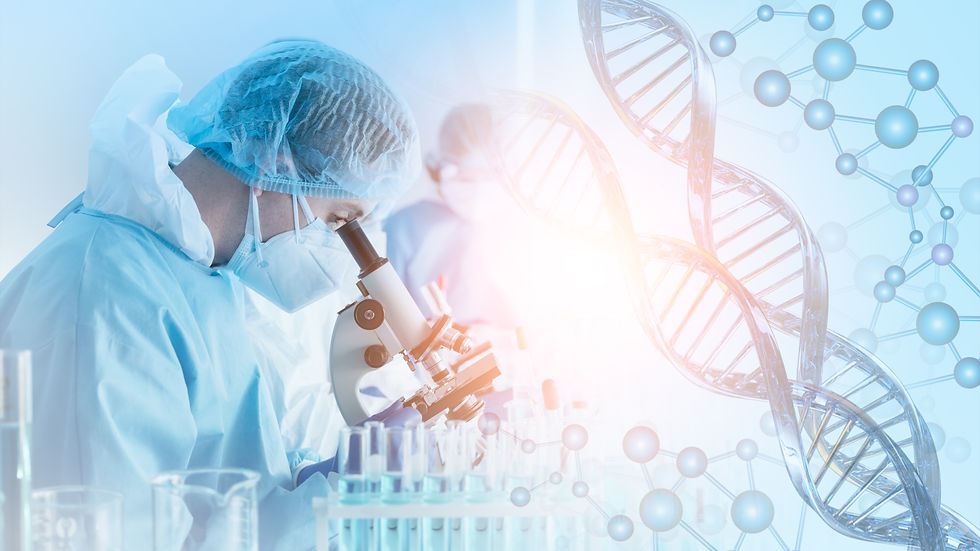
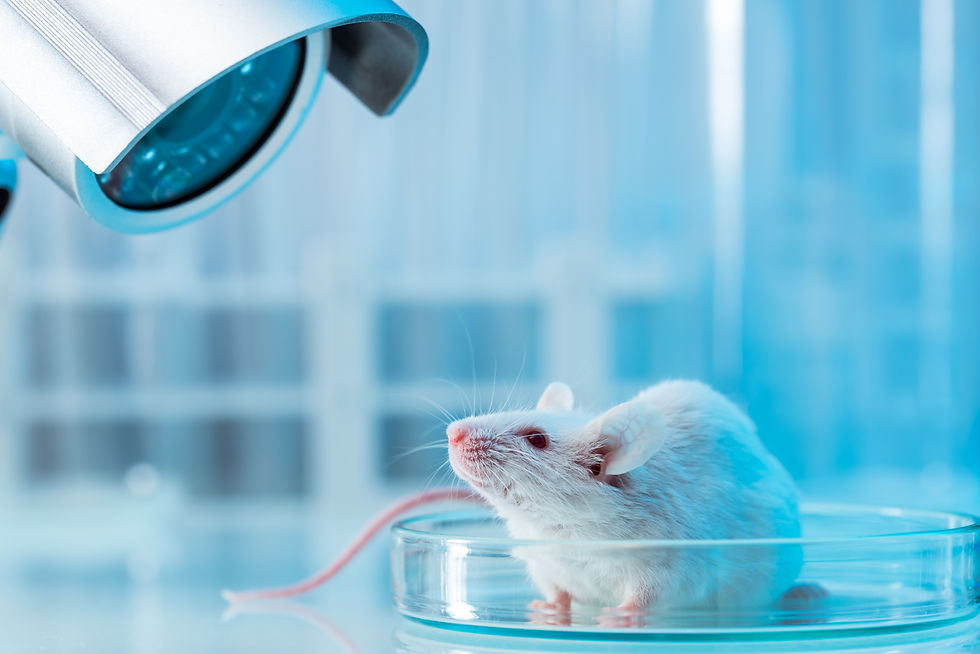
DRUG DISCOVERY PHASE
The AI docking algorithm independently developed by us is superior to traditional algorithms in speed and accuracy (expandable to a molecular library of billions), increasing the accuracy from the current 10% to 60%, and adding quantum descriptors to improve the structural novelty of the generated molecules.
IN VITRO ACTIVE PHASE
The protein conformation flip simulation algorithm independently developed by us can assist experimentalists in optimizing Biomedical Assays and help explore the reaction pathways of interactions between lead compounds and target proteins.
This is the space to describe the product. Write a short overview that includes important features, pricing and other relevant info for a potential buyer. Consider adding an image or video to show off the product and entice visitors to make a purchase.
BIOLOGICAL EXPERIMENT PHASE
By accumulating drugability data for many years, our original RNA sequence analysis technology is used to efficiently explore biological pathways and create a drugability model with high accuracy.
CLINICAL TRIAL PHASE
Using omics and clinical data, we build multimodal models of target-disease and RNA/gene, analyze target-disease relationships, discover unique mature targets, and recommend indications.
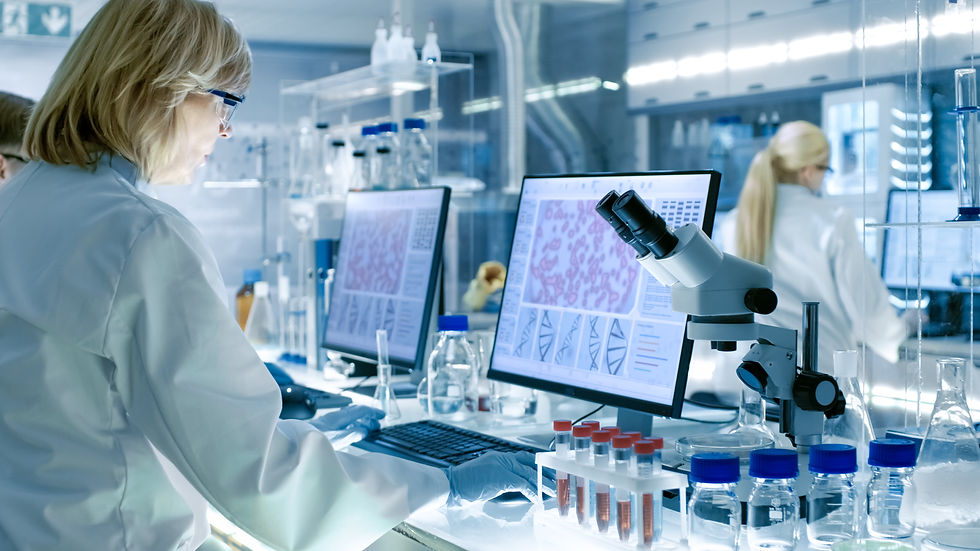
lead the future
Provide New Solutions
The Future of Pharmacy
Technological Progress
Deep Learning and Neural Networks
The success of deep learning in image recognition and natural language processing has been applied to the analysis of medicinal chemistry and biological data. Graph Convolutional Networks (GCNs) are excellent at predicting the properties of chemical molecules.
Natural Language Processing (NLP)
NLP technology is used to extract valuable information from a large body of literature, patents and databases to help researchers keep track of the latest scientific advances.
Combinatorial Chemistry and Quantum Computing
Combinatorial chemistry combines AI techniques for designing and screening large libraries of compounds. Quantum computing is expected to solve the problem of insufficient computing resources in the future.
The Future of Pharmacy
AI Applications
Target Discovery
Use AI to analyze biological data, identify disease-related biomarkers and genes, and thus identify new drug targets. For example, use machine learning models to analyze gene expression data and protein interaction networks.
Drug Screening
Traditional drug screening methods are time-consuming and costly. AI can accelerate the search for potential drug molecules through virtual screening and high-throughput screening techniques. For example, deep learning models can predict the binding affinity of compounds to targets.
Drug Design
AI algorithms can generate molecular structures with specific properties to optimize the chemical properties and biological activity of drugs. For example, generative adversarial networks (GANs) can be used for molecule generation.
Clinical Trial Optimization
AI can help design more efficient clinical trials, optimize trial design, patient recruitment and data analysis, and thus improve trial success rates.